Protein sequence coevolution, energy landscapes and applications to large protein complexes
DIPC Seminars
- Speaker
-
Jose Onuchic
CTBP - Rice University, USA - When
-
2023/05/22
12:00 - Place
- Hybrid Seminar: Donostia International Physics Center
- Host
- Ricardo Díez Muiño
- Add to calendar
-
iCal
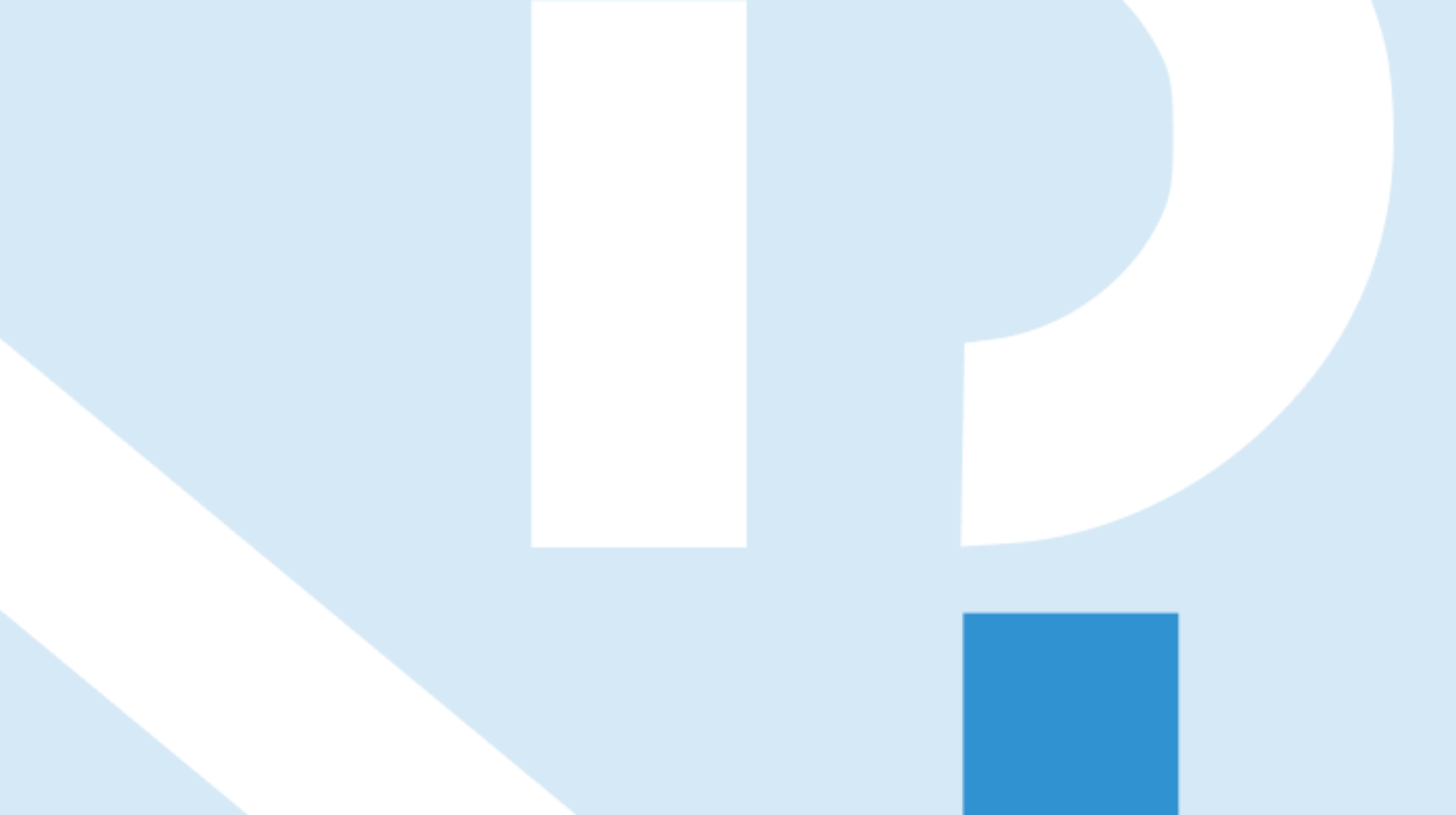
Energy landscape theory has been a powerful approach to study protein folding dynamics and function. The discovery that an accurate estimate of the joint probability distribution of amino acid occupancies in protein families provides insights about residue-residue coevolution and concrete details about protein folding landscapes has also advanced structural biophysics. Our realization that the collection of couplings and local fields as parameters of such distribution is inherently connected with the thermodynamics of
sequence selection towards folding and function demonstrates the importance of coevolutionary methods to understand stability and function of biomolecules. The synergy between structure based models and coevolutionary information has spearheaded the field of
structure prediction, including protein and RNA, as well as accelerating the discovery of functional structural states and the prediction of protein complexes. Coevolution signals can also be used to create protein recognition metrics, which led to successful experimental efforts, and the uncovering of novel molecular interactions. This idea has opened the door to encode recognition in protein pairs.
Recently this approach has been used to predict extremely large protein assemblies consisting of structural maintenance of chromosomes (SMC) and kleisin subunits which are essential for the process of chromosome segregation across all domains of life. While limited
structural data exist for the proteins that comprise the (SMC)–kleisin complex, using an integrative approach combining both crystallographic data and coevolutionary information, we have predicted an atomicscale structure of the whole condensing complex in
prokaryotes.
These ideas from co-evolution can also be utilized in genome folding and function. The energy landscape of the model was derived by using the maximum entropy principle and relies on two experimentally derived inputs: a classification of loci into chromatin types and a catalog of the positions of chromatin loops. This model was generalized by utilizing a neural network to infer these chromatin types using epigenetic marks present at a locus, as assayed by ChIP-Seq. The ensemble of structures resulting from these simulations completely agree with HI-C data and exhibits unknotted chromosomes, phase separation of chromatin types, and a tendency for open chromatin to lie at the periphery of chromosome territories.
* supported by the NSF and the Welch Foundation
Zoom: https://dipc-org.zoom.us/j/99563711582
Youtube: https://youtube.com/live/Ba0JZJTex7E