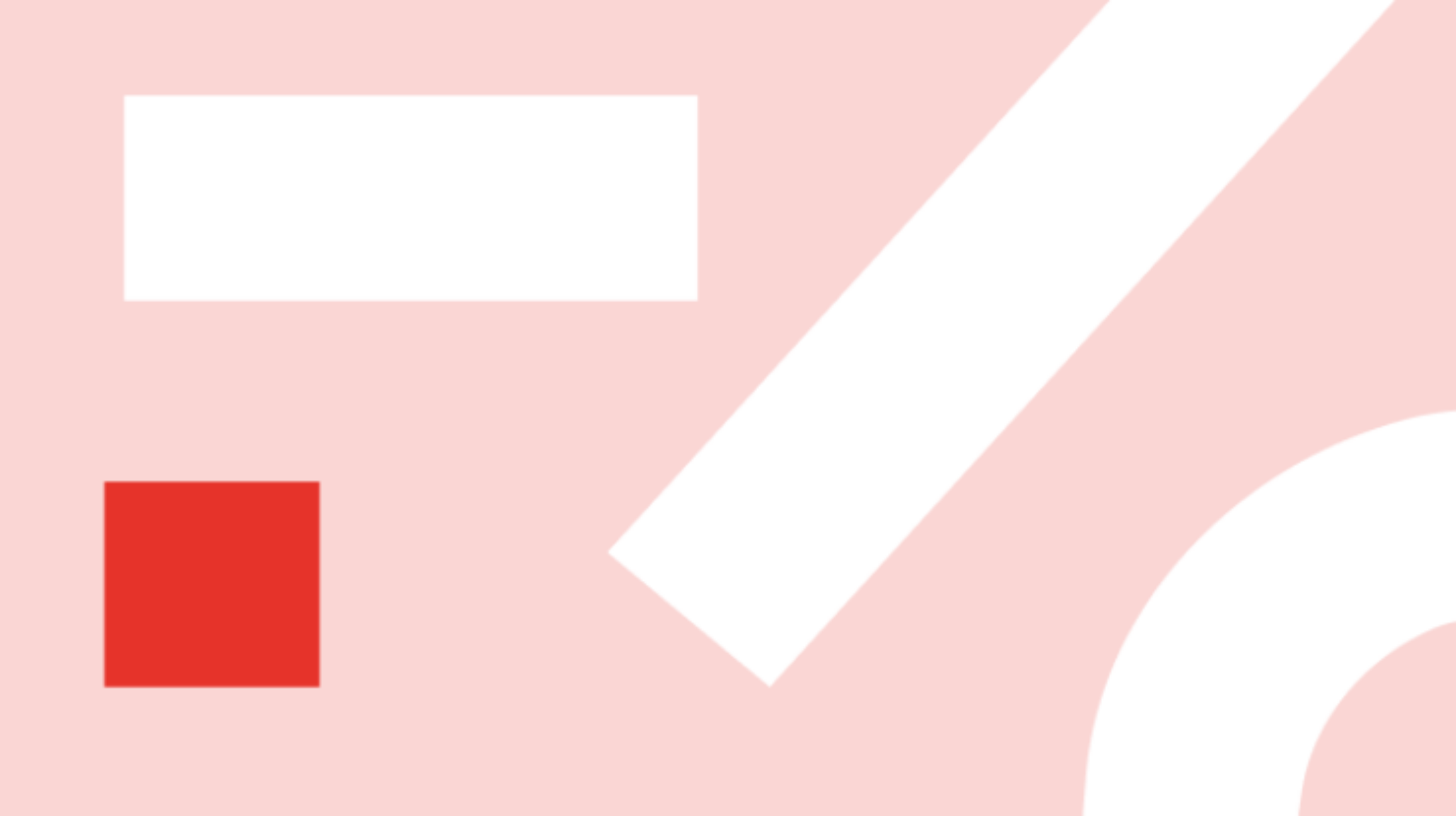
Fast and high-precision qubit control is crucial for new quantum technologies,
including quantum computing, quantum sensing, quantum machine learning. In
this talk, I will introduce the recent progress of quantum control and quantum
computing algorithms which we have achieved with the application of the
techniques of Shortcuts to Adiabaticity (STA) and machine learning. We
integrate STA in the design of the dynamical decoupling sequences that drives
the interaction between the sensor and target signals, and achieve the error-
resilient scheme [1]. Deep Reinforcement Learning (DRL) leads to robust
digital quantum control with robustness against systematic errors [2]. The
experiment [3] verifies the efficiency of the protocol and reveals a general
framework of digital quantum control. Neural networks enable to characterize
targets with minimal knowledge of the physical model, in regimes where the
quantum sensor presents complex responses under the shot noise due to a
reduced number of measurements [4]. We also propose a speed-up quantum
perceptron [5] with STA where the control field on the perceptron is inversely
engineered leading to a rapid nonlinear response with a sigmoid activation
function. The above results show that the combination of STA and machine
learning is helpful to design feasible speed-up protocols for quantum control.
References:
[1] C. Munuera-Javaloy, Y. Ban, X. Chen, and J. Casanova, Robust Detection of
High-Frequency Signals at the Nanoscale, Phys. Rev. Applied 14, 054054 (2020).
[2] Y. Ding, Y. Ban, J. D. Martin-Guerrero, E. Solano, J. Casanova, and X.
Chen, Breaking Adiabatic Quantum Control with Deep Learning, arXiv:
2009.04297.
[3] M. Ai, Y. Ding, Y. Ban, J. D. Martin-Guerrero, J. Casanova, J. Cui, Y.
Huang, X. Chen, C.-F. Li, and G.-C Guo, Experimentally Realizing Efficient
Quantum Control with Reinforcement Learning, arXiv: 2101.09020.
[4] Y. Ban, J. Echanobe, Y. Ding, R. Puebla, and J. Casanova, Neural-network-
based parameter estimation for quantum detection, arXiv: 2012.07677.
[5] Y. Ban, X. Chen, E. Torrontegui, E. Solano, and J. Casanova, Speed-up
Quantum Perceptron via Shortcuts to Adiabaticity, arXiv: 2003.09938.
Host: Roman Orus
ZOOM: [https://dipc-org.zoom.us/j/85748995214](https://youtu.be/MBthv36PMag)
YouTube: [https://youtu.be/MBthv36PMag](https://youtu.be/MBthv36PMag)