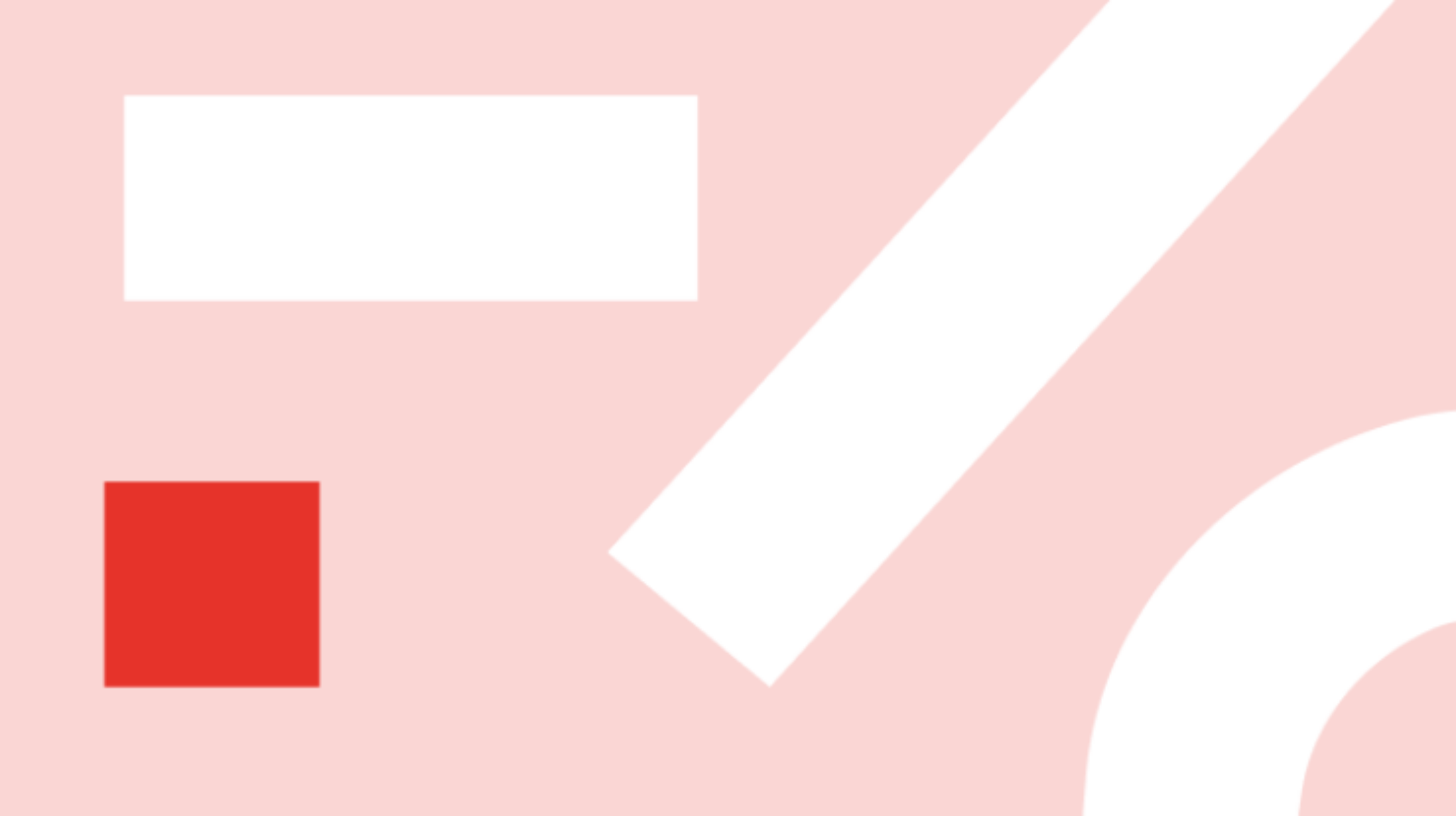
AI is driving a paradigm shift in various social and scientific areas,
including condensed matter physics and materials science. Given the large and
growing amount of information provided in computational databases (millions of
entries) [1] and high-resolution experiments [2, 3], automatic analytical
tools need to be developed. In this talk, we use machine learning to address a
crucial step in the characterization of any material: the classification of
its crystal geometry. Specifically, we introduce ARISE [4], a crystal-
structure identification method based on Bayesian deep learning. As a major
step forward, ARISE is robust to structural noise and can treat – in
automatic fashion - more than 100 crystal structures, a number that can be
extended on demand. While being trained on ideal structures only, ARISE
correctly characterizes strongly perturbed single- and polycrystalline
systems, from both synthetic and experimental resources. The probabilistic
nature of the Bayesian deep-learning model allows to obtain principled
uncertainty estimates. Notably, these are found to be correlated with
crystalline order, which is demonstrated by analyzing crystal growth in
experimental, time-resolved measurements of metallic nanoparticles. We also
discuss a connection to unsupervised learning, allowing exploration of
materials dataspaces: the neural-network model automatically learns data
representations that contain information on structurally diverse crystal
geometries. Using state-of-the-art clustering, physically meaningful subgroups
can be identified in the neural-network latent space, which are shown, e.g.,
to correspond to distinct, experimentally verified grain-boundary phases [3].
Moreover, dimension- reduction analysis allows us to create low-dimensional,
interpretable materials charts that visualize complex (i.e., single-, poly-,
quasi-crystalline and amorphous) data from both theoretical and experimental
origin [2, 3]. This work enables the hitherto hindered analysis of noisy
atomic structural data from computations or experiments.
[1] C. Draxl, M. Scheffler, MRS Bull. 43, 676-682 (2018)
[2] Y. Yang et al. Nature 592, 60 (2021)
[3] T. Meiners, T. Frolov, R.E. Rudd, et al. Nature 579, 375– 378 (2020)
[4] A. Leitherer, A. Ziletti and L. M. Ghiringhelli. Nat. Commun. 12, 6234
(2021)
Host: Roma Orus