Improving cosmological simulations: semi-analytic models, the internal structure of haloes, and machine learning techniques
PhD Program
- Speaker
-
Daniel López Cano
- When
-
2024/09/06
11:00 - Place
- Faculty of Science, Autonomous University of Madrid
- Add to calendar
-
iCal
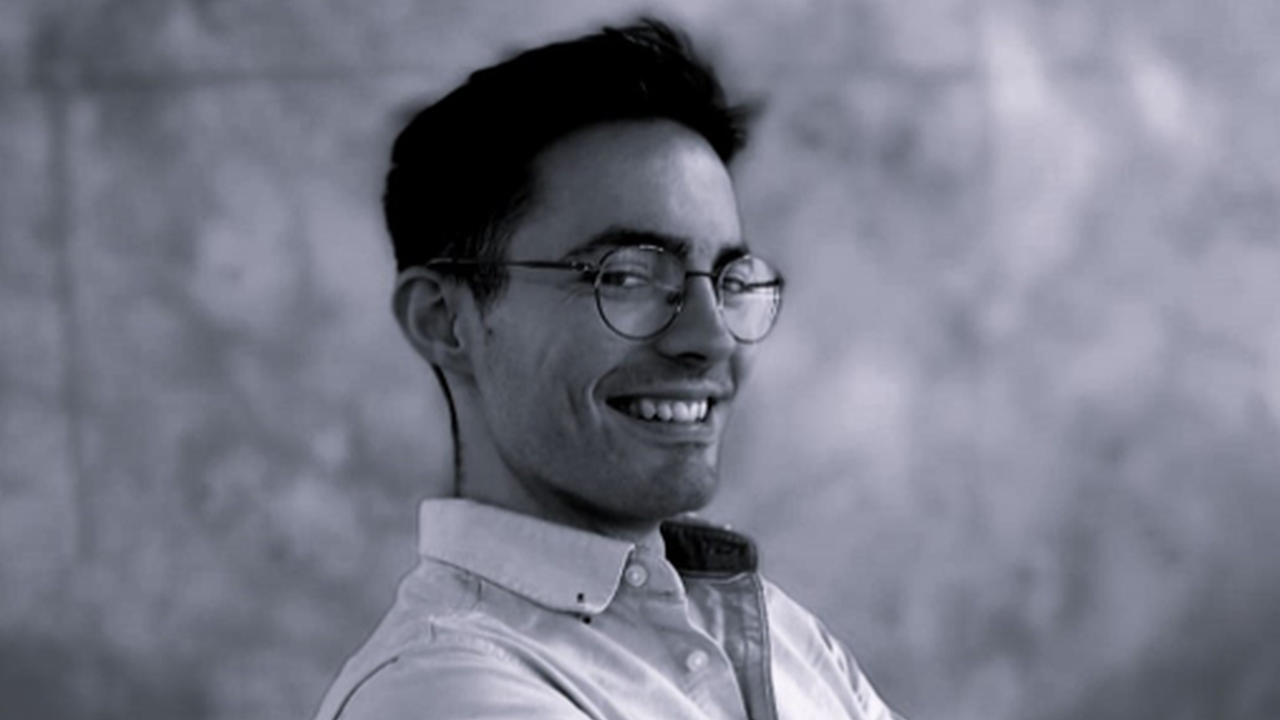
PhD Thesis defense by Daniel López Cano
Supervisors: Raúl Esteban Angulo de la Fuente (DIPC, Ikerbasque)
Astrophysics - Computational Cosmology
This thesis compiles three scientific articles developed during my doctoral studies in computational cosmology, focusing on the analysis and interpretation of cosmological simulations. These simulations model the gravitational interactions of particles to study structure formation and connect theoretical predictions with galaxy observations.
First, I explore how semi-analytical models can populate gravity-only simulations with galaxies, bridging the gap between simulations and telescope observations. Second, I present a model for predicting the internal structure of dark matter halos, capturing how their properties depend on formation time, mass, and the assumed cosmological model. Finally, I introduce a machine learning-based model that identifies regions in the initial conditions of simulations that lead to the formation of dark matter halos, offering insights into halo formation processes.
These studies enhance the interpretation of cosmological simulations and demonstrate how machine learning complements traditional methods in structure formation research. The findings contribute to our understanding of the Universe and provide a novel approach to observational cosmology.